Program
Invited Talk
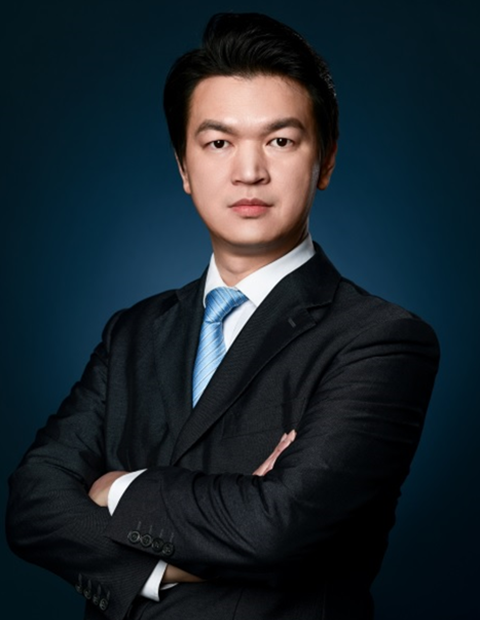
Jun Wu
Professor,Shanghai Jiao Tong University
Abstract | Digital twin has expanded a broad space for accelerating digital transformation and intelligent upgrading around the world, supporting important fields such as smart cities, smart ocean and air, smart manufacturing, etc. Trust and smart management for the resources and knowledge is the important topic in digital twin. To address this, the blockchian and artificial empowered digital twin will be discussed in this talk. Firstly, the architecture of blockchian based digital twin as a service will be presented. Secondly, blockchian based knowledge sharing approach in digital twin will be shown. Finally, deep reinforcement learning will introduced in digital twin for coordinate the computing, communication and control resources in trusted digital twin. |
---|---|
Biography | Jun Wu is a professor of School of Electronic Information and Eletrical Engineering, Shanghai Jiao Tong University, China. His research interests include the networking technologies and security for Internet of Things, cloud computing/fog computing, big data, etc. He has published more than 190 papers in international journals and conference, such as T-IFS, TDSC, TII, TMC, AAAI, etc. He is the chair of IEEE 21451 standard working group for smart sensors. He got the many best awards, such as 2019 IEEE TETC best paper, TSP 2019 best paper, 2018 best conference paper of IEEE ComSoc Technical Committee on CSIM, etc. Also, he got the The Second Award for Natural Science of The Chinese Ministry of Education in 2019 and the Second Award for Science Development of Chinese Institute of Electronics in 2018. |
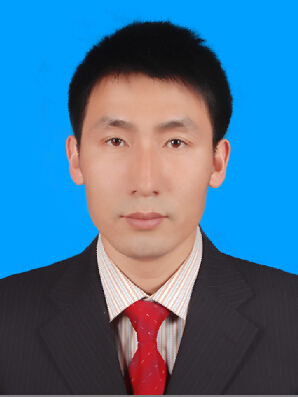
Tie Qiu
Professor,TianJin University
Abstract | Blockchain has been utilized to manage distributed multi-cloud storage in the Industrial Internet of Things(IIoT). Existing approaches commonly use trusted third-party servers or middle-wares to search data allocation strategies and use blockchain to enhance security. However, finding a fair data allocation strategy is hard when the third-party brokers are manipulated. In this talk, a scalable two-layer blockchain system is presented. |
---|---|
Biography | Dr. Tie Qiu is currently a Full Professor at School of Computer Science and Technology, Tianjin University, China. Prior to this position, he held assistant professor in 2008 and associate professor in 2013 at School of Software, Dalian University of Technology. He was a visiting professor at department of electrical and computer engineering of Iowa State University in U.S. (2014-2015). He serves as an associate editor of IEEE/ACM Transactions on Networking (ToN), IEEE Transactions on Network Science and Engineering (TNSE) and IEEE Transactions on Systems, Man, and Cybernetics: Systems, area editor of Ad Hoc Networks (Elsevier), associate editor of Computers and Electrical Engineering (Elsevier), Human-centric Computing and Information Sciences (Springer), a guest editor of Future Generation Computer Systems. He serves as General Chair, Program Chair, Workshop Chair, Publicity Chair, Publication Chair or TPC Member of a number of international conferences. He has authored/co-authored 10 books, over 200 scientific papers in international journals and conference proceedings, such as IEEE/ACM Transactions on Networking, IEEE Transactions on Mobile Computing, IEEE Transactions on Knowledge and Data Engineering, IEEE Transactions on Industrial Informatics, IEEE Communications Surveys & Tutorials, IEEE Communications, INFOCOM, GLOBECOM etc. There are 18 papers listed as ESI highly cited papers. He has contributed to the development of 6 copyrighted software systems and invented 20 patents. He is a distinguished member of China Computer Federation (CCF) and a Senior Member of IEEE and ACM. |
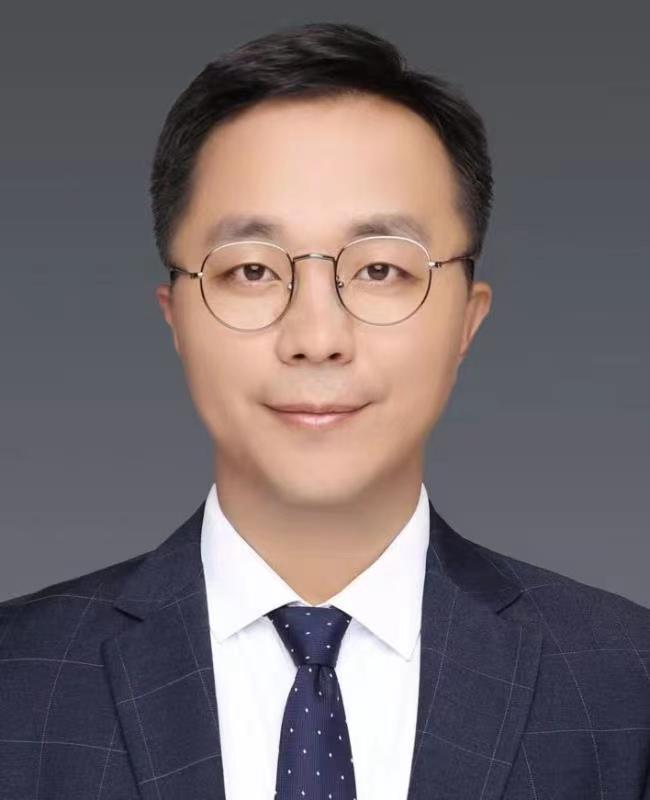
Zhuo Ma
Professor,Xidian University
Learn to Forget: Making AI More Secure via Machine Unlearning
Abstract | Machine learning is a popular technique used for finding valued info from massive amount of data.However, since most training sets are collected publicly, it is inevitable that some public data resources may contain poison data crafted by the adversary. Besides potentially leading negative effect on model performance, these unexpected data can also leave backdoors for the adversary to control the behavior of trained models. In this report, we present a new direction to mitigate the side-effect of poison data, which is called machine unlearning, a reversed technique to machine learning. Starting from the point of essential concept, we introduce what is machine unlearning, and then, analyze how to achieve machine unlearning efficiently. Finally, an example is presented to show the application of machine unlearning to implement backdoor defense. |
---|---|
Biography | Zhuo Ma received his Ph.D. degree in Computer Architecture from Xidian University, Xi'an, China, in 2010. Now, he is a professor at school of Cyber Engineering, Xidian University. He awards "Huashan Scholars" Distinguished Professor. His research interests include cryptography, AI security, and IoT security. |
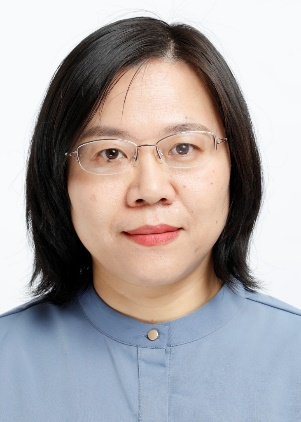
Liping Qian
Professor,Zhejiang University of Technology
Distributed Charging-Record Management for Electric Vehicle Networks via Blockchain
Abstract | The deep penetration of electric vehicles (EVs) into the transportation section and the associated charging management has yielded a critical issue, namely, how to efficiently store the generated charging records. In this talk, we investigate the cost-efficient charging-record storage scheme by exploiting blockchain (BC). Accounting for the operational cost due to the consensus process via the practical Byzantine fault tolerance (PBFT) protocol, we model the associated cost for storing the charging records via an ideal multi-blockchain system and formulate a joint optimization of the storage selection (i.e., either storing the charging record locally or selecting one of the BCs for storing the charging record) and server-node allocation for each BC, with the objective of minimizing a system-wise cost. Then, we exploit the decomposition structure and propose a layered algorithm (i.e., the bottom subproblem for determining the optimal storage selection and the top problem for finding the server-node allocation) to solve it. For the bottom subproblem, we exploit the nature of minimum weighted matching of the problem and propose a distributed auction-based algorithm for computing the optimal storage selection. With the optimal solution from the subproblem, we further propose an annealing-based algorithm to determine the server-node allocation for each BC. |
---|---|
Biography | Liping Qian (Senior Member, IEEE) received the Ph.D. degree in information engineering from The Chinese University of Hong Kong, Hong in 2010. During 2010–2011, she was a Postdoctoral Research Associate with The Chinese University of Hong Kong. Since 2011, she has been with the College of Information Engineering, Zhejiang University of Technology, Hangzhou, China, where she is currently a Full Professor. From 2016 to 2017, she was a Visiting Scholar with the Broadband Communications Research Group, ECE Department, University of Waterloo, Waterloo, ON, Canada. Her research interests include wireless communication and networking, resource management in wireless networks, massive IoTs, mobile edge computing, emerging multiple access techniques, and machine learning oriented towards wireless communications. She was the corecipient of the IEEE Marconi Prize Paper Award in Wireless Communications in 2011, Best Paper Award from IEEE ICC 2016, Best Paper Award from IEEE Communication Society GCCTC 2017, and Best Paper Award from the Digital Communications and Networking. She is currently on the Editorial Board of IET Communications. |
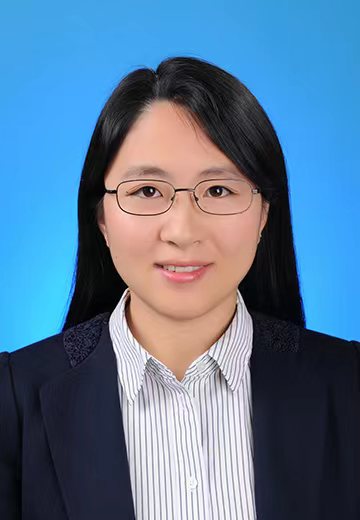
Yuan Liu
Professor,Guangzhou University
Proof of Learning (PoLe): Machine Learning Powered Blockchain Consensus Mechanism
Abstract | The progress of deep learning (DL), especially the recent development of automatic design of networks, has brought unprecedented performance gains at heavy computational cost. On the other hand, blockchain systems routinely perform a huge amount of computation that does not achieve practical purposes in order to build Proof-of-Work (PoW) consensus from decentralized participants. In this study, we propose a new consensus mechanism, Proof of Learning (PoLe), which directs the computation spent for consensus toward optimization of neural networks (NN). In our mechanism, the training/testing data are released to the entire blockchain network (BCN) and the consensus nodes train NN models on the data, which serves as the proof of learning. We experimentally compare the PoLe protocol with Proof of Work (PoW) and show that PoLe can achieve a more stable block generation rate, which leads to more efficient transaction processing. We also introduce a novel cheating prevention mechanism, Secure Mapping Layer (SML), which can be straightforwardly implemented as a linear NN layer. Empirical evaluation shows that SML can detect cheating nodes at small cost to the predictive performance. |
---|---|
Biography | Yuan Liu a professor at Cyberspace Institute of Advanced Technology of Guangzhou University in Guangdong, China. She is Committee of CCF Blockchain Commission, And Member of Blockchain Committee of China Communication Society. She achieved her Ph.D degree in School of Computer Engineering from Nanyang Technological University (NTU), Singapore, in 2014. She received her B.Sc degree in the honor school, Harbin Institute of Technology, China, in 2010. She was an associate professor at northeastern university, China from 2015 to 2021. From 2014 to 2015, she ever worked as Research Fellow at Joint NTU-UBC Research Centre of Excellence in Active Living for the Elderly (LILY), NTU, Singapore. |
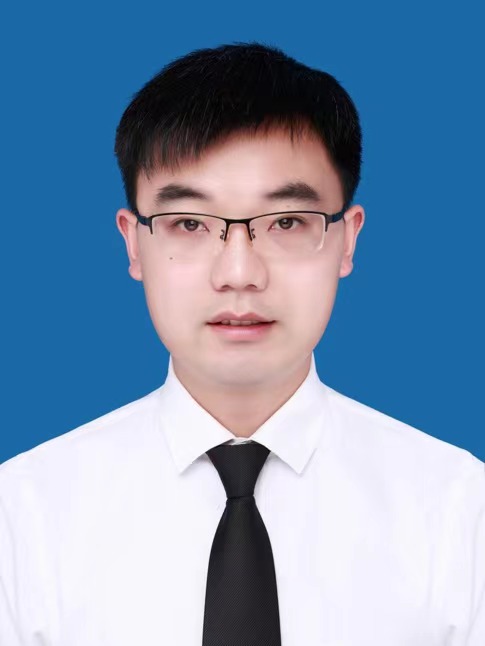
Ju Ren
Associate Professor ,Tsinghua University
Add Your Heading Text HereEfficient and Continual Federated Meta-Learning under Data and System Heterogeneity
Abstract | Federated learning enables distributed mobile devices to collaboratively learn a shared model without exposing their raw data, which mitigates the risk of privacy leakage and promotes mutual benefits between devices. However, the diverse data distribution among devices, i.e., data heterogeneity, usually results in significant performance degradation. The problem comes from that a globally shared model can hardly achieve the balance between personalization and generalization. To address this challenge, I will introduce our recent studies on federated meta-learning, where mobile devices can collaboratively learn a meta-model, aided by the knowledge transfer from prior tasks to keep personalization and continuous learning for local model. This talk will also bring several novel techniques we designed to conquer the challenges in deploying federated meta-learning on resource-constrained devices, including how to alleviate the computation burden, and how to jointly optimize client selection and communication resource allocation for fast model convergence. |
---|---|
Biography | Ju Ren received the B.Sc. (2009), M.Sc. (2012), Ph.D. (2016) degrees all in computer science, from Central South University, China. Currently, he is an associate professor with the Department of Computer Science and Technology, Tsinghua University, China. His research interests include Internet-of-Things, edge computing, as well as network security and privacy. He currently serves as an associate editor for IEEE Transactions on Vehicular Technology and Peer-to-Peer Networking and Applications. He also served as the general co-chair for IEEE BigDataSE’20, the TPC co-chair for IEEE BigDataSE’19, poster co-chair for IEEE MASS’18, track co-chair for IEEE/CIC ICCC’19, IEEE I-SPAN’18 and VTC’17 Fall, etc. He received many best paper awards from IEEE flagship conferences, including IEEE ICC’19 and IEEE HPCC’19, etc., the IEEE TCSC Early Career Researcher Award (2019), and the IEEE ComSoc Asia-Pacific Best Young Researcher Award (2021). He was recognized as a highly cited researcher by Clarivate (2020 & 2021). |
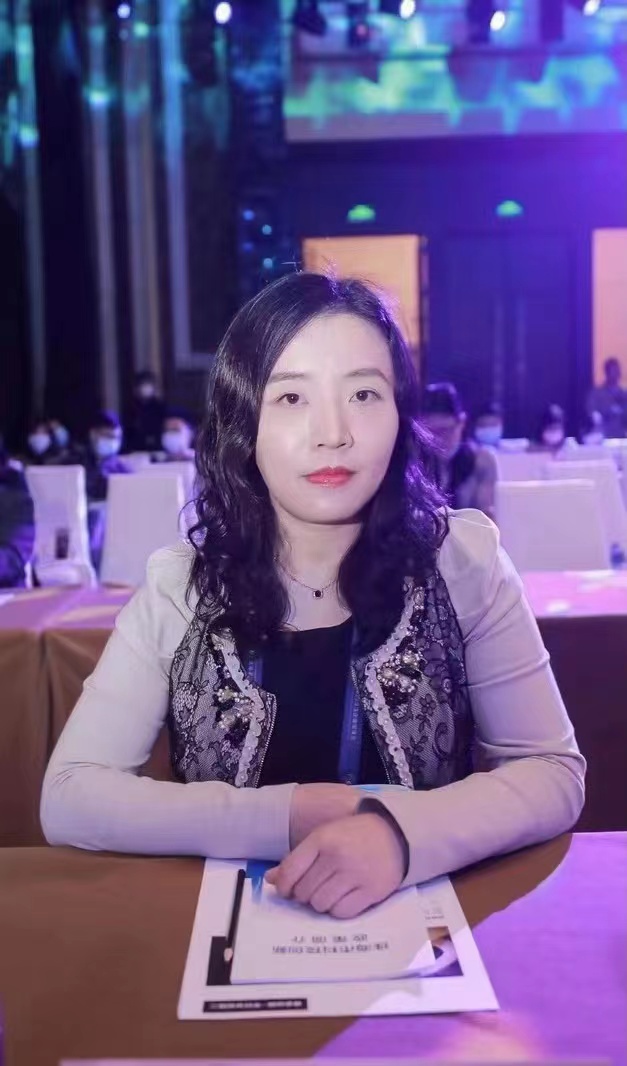
Jing Lu
Blockchain Chief Scientist of YGSoft Inc
Digital ID Applications Based on Blockchain
Abstract | Globally speaking, more and more countries are promoting the digital government strategy, taking data as the centre, participating to digital ID constructions. In this presentation, we will share our digital ID technical solutions based on blockchain, including the business model for government digital ID applications, the overall architecture and open capabilities of the digital ID platform, the core architecture of the underlying blockchain platform, as well as the application cases of the digital ID based on blockchain. |
---|---|
Biography | Lu Jing (1981-), Ph.D., zhuhai high-level personnel. Currently, she is the chief scientist of Blockchain of YGSoft Inc., and the external master supervisor of Central China Normal University and Zhongnan University of Economics and Law. Since 2015, she has been engaged in the development of blockchain platform and products in YGSoft Inc., and completed a number of government projects. She has also published two monographs, over 60 blockchain invention patents (in which 21 as the first inventor, 7 have been authorized), and more than 20 SCI and EI journal papers. |